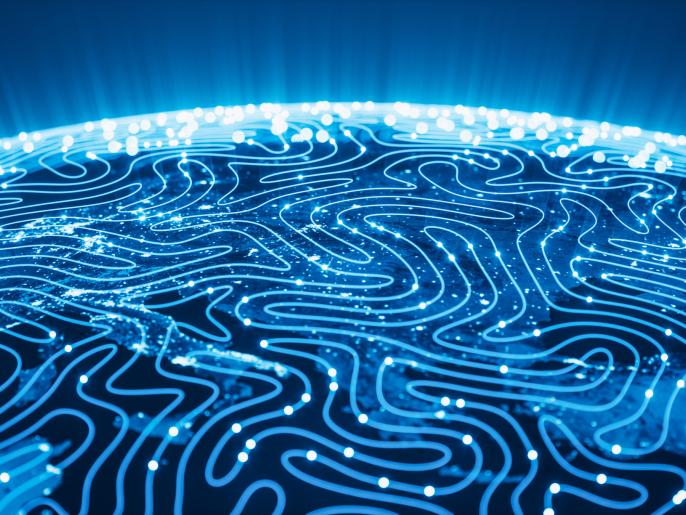
Workshop on Mitigating AI Bias in Context
Automated decision-making is appealing because AI/ML systems produce more consistent, traceable, and repeatable decisions compared to humans; however, these systems come with risks that can result in discriminatory outcomes. For example, unmitigated bias that manifests in AI/ML systems used to support automated decision-making in consumer and small business credit underwriting can lead to unfair results, causing harms to the individual applicants and potentially rippling throughout society, leading to distrust of AI-based technology and institutions that rely on them. AI/ML-based credit underwriting decision technologies and the models and datasets that underlie them create transparency challenges generally and those raise particular concerns about identification and mitigation of bias in enterprises that seek to use machine learning in their credit underwriting pipeline. Yet ML models tend to exhibit “unexpectedly poor behavior when deployed in real world domains” without domain-specific constraints supplied by human operators, as discussed in NIST Special Publication (SP) 1270, Towards a Standard for Identifying and Managing Bias in Artificial Intelligence. Similar problems exist in other contexts, such as hiring and school admissions.
The heavy reliance on proxies can also be a significant source of bias in AI/ML applications. For example, in credit underwriting an AI system might be developed using input variables such as “length of time in prior employment,” which might disadvantage candidates who are unable to find stable transportation, as a measurable proxy in lieu of the not directly measurable concept of “employment suitability.” The algorithm might also include a predictor variable such as residence zip code, which may relate to other socio-economic factors, and may result in ranking certain groups lower in desirability for credit approval. This in turn would cause AI/ML systems to contribute to biased outcomes. Similar issues exist in other contexts. For further information about how the use of proxies may lead to negative consequences in other contexts, see NIST SP 1270.